
Книги по МРТ КТ на английском языке / Functional Neuroimaging in Child Psychiatry Ernst 1 ed 2000
.pdf
24 P. Herscovitch and M. Ernst
Huang, S. C., Phelps, M. E., HoVman, E. J., Sideris, K., Selin, C. J. and Kuhl, D. E. (1980). Non-invasive determination of local cerebral metabolic rate of glucose in man. Am. J. Physiol., 238, E69±82.
Huang, S. C., Phelps, M. E., HoVman, E. J. and Kuhl, D. E. (1981). Error sensitivity of Xuorodeoxyglucose method for measurement of cerebral metabolic rate of glucose. J. Cerebr. Blood Flow Metab., 1, 391±401.
Huda, W. and Scrimger, J. W. (1989). Irradiation of volunteers in nuclear medicine. J. Nucl. Med., 30, 260±4.
Iida, H., Rhodes, C. G., de Silva, R. et al. (1992). Use of the left ventricular time±activity curve as a noninvasive input function in oxygen-15-water positron emission tomography. J. Nucl. Med.,
33, 1669±77.
Jacobs, A., Put, E., Ingels, M. and Bossuyt, A. (1994). Prospective evaluation of technetium-99m-HMPAO SPECT in mild and moderate traumatic brain injury. J. Nucl. Med., 35, 942±7.
Jueptner, M. and Weiller, C. (1995). Does measurement of regional cerebral blood Xow reXect synaptic activity ± implications for PET and fMRI. [Review] Neuroimage 2, 148±56.
Juni, J. E. (1994). Taking brain SPECT seriously: reXections on recent clinical reports in the Journal of Nuclear Medicine. J. Nucl. Med., 35, 1891±5.
Karp, J. S., Daube-Witherspoon, M. E., HoVman, E. J. et al. (1991). Performance standards in positron emission tomography. J. Nucl. Med., 32, 2342±50.
Kassis, A. I. (1992). The MIRD approach: remembering the limitations. J. Nucl. Med., 33, 781±2.
Kety, S. S. (1951). The theory and applications of the exchange of inert gas at the lungs and tissues. Pharmacol. Rev., 3, 1±41.
Kilbourn, M. (1991). Radiotracers for PET studies of neurotransmitter binding sites: design considerations. In InVivo Imaging of Neurotransmitter Functions in Brain, Heart and Tumors, ed. D. E. Kuhl, pp. 47±65. Washington, DC: American College of Nuclear Physicians.
Koeppe, R. A. and Hutchins, G. D. (1992). Instrumentation for positron emission tomography: tomographs and data processing and display systems. Semin. Nucl. Med., 22, 162±81.
Koeppe, R. A., Holden, J. E. and Ip, W. R. (1985). Performance comparison of parameter estimation techniques for the quantitation of local cerebral blood Xow by dynamic positron computed tomography. J. Cereb. Blood Flow Metab., 5, 224±34.
Koeppe, R. A., HolthoV, V. A., Frey, K. A., Kilbourn, M. R. and Kuhl, D. E. (1991). Compartmental analysis of [11C]Xumazenil kinetics for the estimation of ligand transport rate and receptor distribution using positron emission tomography. J. Cereb. Blood Flow Metab., 11, 735±44.
Kuhl, D. E., Alavi, A., HoVman, E. J. et al. (1980). Local cerebral blood volume in head-injured patients: determination by emission computed tomography of 99mTc-labeled red cells. J. Neurosurg., 52, 309±20.
Lammertsma, A. A., Jones, T., Frackowiak, R. S. J. and Lenzi, G.-L. (1981). A theoretical study of the steady-state model for measuring regional cerebral blood Xow and oxygen utilization using oxygen-15. J. Comput. Assist. Tomogr., 5, 544±50.
Lammertsma, A. A., Wise, R. J. S., Heather, J. D. et al. (1983). Correction for the presence of intravascular oxygen-15 in the
steady-state technique for measuring regional oxygen extraction ratio in the brain. 2. Results in normal subjects and brain tumor and stroke patients. J. Cereb. Blood Flow Metab., 3, 425±31.
Lammertsma, A. A., Brooks, D. J., Beaney, R. P. et al. (1984). In vivo measurement of regional cerebral haematocrit using positron emission tomography. J. Cereb. Blood Flow Metab., 4, 317±22.
Lammertsma, A. A., Brooks, D. J., Frackowiak, R. S. J. et al. (1987). Measurement of glucose utilization with [18F]2-Xuoro-2-deoxy-
D-glucose: a comparison of diVerent analytical methods. J. Cereb. Blood Flow Metab., 7, 161±72.
Landau, W. M., Freygang, W. H., Jr, Rowland, L. P., SokoloV, L. and Kety, S. (1955). The local circulation of the living brain; values in the unanesthetized and anesthetized cat. Trans. Am. Neurol. Assoc., 80, 125±9.
Laruelle, M., Baldwin, R. M., Rattner, Z. et al. (1994a). SPECT quantiWcation of [123I]iomazenil binding to benzodiazepine receptors in nonhuman primates. I. Kinetic modeling of single bolus experiments. J. Cereb. Blood Flow Metab., 14, 439±52.
Laruelle, M., van, Dyck, C., Abi, D. A. et al. (1994b). Compartmental modeling of iodine-123-iodobenzofuran binding to dopamine D2 receptors in healthy subjects. J. Nucl. Med., 35, 743±54.
Leenders, K. L., Perani, D., Lammertsma, A. A. et al. (1990). Cerebral blood Xow, blood volume and oxygen utilization: normal values and eVect of age. Brain, 113, 27±47.
Leniger-Follert, E. and Hossman, K.-A. (1979). Simultaneous measurements of microXow and evoked potentials in the somatomotor cortex of rat brain during speciWc sensory activation.
PXugers Arch., 380, 85±9.
Lockwood, A. H. (1985). Invasiveness in studies of brain function by positron emission tomography. J. Cereb. Blood Flow Metab., 5, 487±9.
Loevinger, R., Budinger, T. F. and Watson, E. E. (1988). MIRD Primer for Absorbed Dose Calculations. New York: Society of Nuclear Medicine.
Lou, H. C., Edvinsson, L. and MacKenzie, E. T. (1987). The concept of coupling blood Xow to brain function: revision required? Ann. Neurol., 22, 289±97.
Martin, W. R. W., Powers, W. J. and Raichle, M. E. (1987). Cerebral blood volume measured with inhaled C15O and positron emission tomography. J. Cerebr. Blood Flow Metab., 7, 421±6.
Masdeu, J. C., Brass, L. M., Holman, B. L. and Kushner, M. J. (1994). Brain single-photon emission computed tomography.
Neurology, 44, 1970±7.
Matthew, E., Andreason, P., Carson, R. E. et al. (1993). Reproducibility of resting cerebral blood-Xow measurements with H215O positron emission tomography in humans. J. Cereb. Blood Flow Metab., 13, 748±54.
Mazziotta, J. C., Phelps, M. E., Plummer, D. and Kuhl, D. E. (1981). Quantitation in positron computed tomography. 5. Physicalanatomical eVects. J. Comput. Assist. Tomogr., 5, 734±43.
McIntosh, A. R., Grady, C. L., Ungerleider, L. G., Haxby, J. V., Rapoport, S. I. and Horwitz, B. (1994). Network analysis of cortical visual pathways mapped with PET. J. Neurosci., 14, 655±66.
Meltzer, C. C., Zubieta, J. K., Links, J. M., Brakeman, P., Stumpf, M. J. and Frost, J. J. (1996). MR-based correction of brain PET
Functional brain imaging with PET/SPECT |
25 |
|
|
measurements for heterogeneous gray-matter radioactivity distribution. J. Cereb. Blood Flow Metab., 16, 650±8.
Minoshima, S., Berger, K. L., Lee, K. S. and Mintun, M. A. (1992). An automated method for rotational correction and centering of three-dimensional functional brain images. J. Nucl. Med., 33, 1579±85.
Mintun, M. A., Raichle, M. E., Martin, W. R. W. and Herscovitch, P. (1984). Brain oxygen utilization measured with O-15 radiotracers and positron emission tomography. J. Nucl. Med., 25, 177±87.
Murase, K., Tanada, S., Fujita, H., Sakaki, S. and Hamamoto, K. (1992). Kinetic behavior of technetium-99m-HMPAO in human brain and quantiWcation of cerebral blood Xow using dynamic SPECT. J. Nucl. Med., 33, 135±43.
Muzik, O., Chugani, D. C., Shen, C. and Chugani, H. T. (1998). Noninvasive imaging of serotonin synthesis rate using PET and
'-methyltryptophan in autistic children. In Quantitative Functional Brain Imaging with Positron Emission Tomography, ed. R. E. Carson, M. E. Daube-Witherspoon and P. Herscovitch, pp. 201±6. San Diego, CA: Academic Press.
Nakai, H.,Yamamoto,Y. L., Diksic, M. et al. (1987). Time-dependent changes of lumped and rate constants in the deoxyglucose method in experimental cerebral ischemia. J. Cereb. Blood Flow Metab., 7, 640±8.
Obrist, W. D., LangWtt, T. W., Jaggi, J., Cruz, J. and Gennarelli, T. A. (1984). Cerebral blood Xow and metabolism in comatose patients with acute head injury. J. Neurosurg., 61, 241±53.
Ohta, S., Meyer, E., Thompson, C. J. and Gjedde, A. (1992). Oxygen consumption of the living human brain measured after a single inhalation of positron emitting oxygen. J. Cereb. Blood Flow Metab., 12, 179±92.
Pelizzari, C. A., Chen, G. T. Y., Spelbring, D. R., Weichselbaum, R. R. and Chen, C.-T. (1989). Accurate three-dimensional registration of CT, PET, and/or MR images of the brain. J. Comput. Assist. Tomogr., 13, 20±6.
Perlman, J. M. and Altman, D. I. (1992). Symmetric cerebral blood
Xow in newborns who have undergone successful extracorporeal membrane oxygenation. Pediatrics, 89, 235±9.
Perlmutter, J. S., Powers, W. J., Herscovitch, P., Fox, P. T. and Raichle, M. E. (1987). Regional asymmetries of cerebral blood Xow, blood volume, oxygen utilization and extraction in normal subjects. J. Cereb. Blood Flow Metab., 7, 64±7.
Phelps, M. E. and HoVman, E. J. (1976). Resolution limit of positron cameras. J. Nucl. Med., 17, 757±8.
Phelps, M. E., Huang, S. C., HoVman, E. J., Selin, C., SokoloV, L. and Kuhl, D. E. (1979). Tomographic measurement of local cerebral glucose metabolic rate in humans with (F-18) 2-Xuoro-2-deoxy-
D-glucose: validation of method. Ann. Neurol., 6, 371±88. Powers, W. J. (1988). Positron emission tomography in the evalua-
tion of cerebrovascular disease: clinical applications? In Clinical Neuroimaging, ed. W. H. Theodore, pp. 49±74. New York: Alan R. Liss.
Powers, W. J. (1991). Cerebral hemodynamics in ischemic cerebrovascular disease. Ann. Neurol., 29, 231±40.
Powers, W. J., Berg, L., Perlmutter, J. S. and Raichle, M. E. (1991). Technology assessment revisited: does positron emission tomography have proven clinical eYcacy? Neurology, 41, 1339±40.
Powers, W. J., Rosenbaum, J. L., Dence, C. S., Markham, J. and Videen, T. O. (1998). Cerebral glucose transport and metabolism in preterm human infants. J. Cereb. Blood Flow Metab., 18, 632±8.
Raichle, M. E. (1987). Circulatory and metabolic correlates of brain function in normal humans. In Handbook of Physiology: The Nervous System, ed. V. B. Mountcastle and F. Plum, pp. 643±74. Bethesda, MD: American Physiological Society.
Raichle, M. E. (1991). The metabolic requirements of functional activity in human brain: a positron emission tomography study. In Fuel Homeostatis and the Nervous System, ed. M. Vranic, S. Efendic and C. H. Hollenberg, pp. 1±4. New York: Plenum.
Raichle, M. E., Martin, W. R. W., Herscovitch, P., Mintun, M. A. and Markham, J. (1983). Brain blood Xow measured with intravenous H215O. II. Implementation and validation. J. Nucl. Med., 24, 790±8.
Rajapakse, J. C., Giedd, J. N., Rumsey, J. M., Vaituzis, A. C., Hamburger, S. D. and Rapoport, J. L. (1996). Regional MRI measurements of the corpus callosum: a methodological and developmental study. Brain Dev., 18, 379±88.
Reivich, M., Kuhl, D., Wolf, A. et al. (1979). The [18F]Xuorodeoxyglucose method for the measurement of local cerebral glucose utilization in man. Circ. Res., 44, 127±37.
Reivich, M., Alavi, A., Wolf, A. et al. (1985). Glucose metabolic rate kinetic model parameter determination in humans: the lumped constants and rate constants for [18F]Xuorodeoxyglucose and [11C]deoxyglucose. J. Cereb. Blood Flow Metab., 5, 179±92.
Sasaki, H., Kanno, I., Murakami, M., Shishido, F. and Uemera, K. (1986). Tomographic mapping of kinetic rate constants in the
Xuorodeoxyglucose model using dynamic positron emission tomography. J. Cereb. Blood Flow Metab., 6, 447±54.
Seibyl, J. P., Laruelle, M., van Dyck, C. H. et al. (1996). Reproducibility of iodine-123-(-CIT SPECT brain measurement of dopamine transporters. J. Nucl. Med., 37, 222±8.
SokoloV, L. (1961). Local cerebral circulation at rest and during altered cerebral activity induced by anesthesia or visual stimulation. In Regional Neurochemistry, ed. S. S. Kety and J. Elkes, pp. 107±17. New York: Pergamon Press.
SokoloV, L. (1981). Relationships among local functional activity, energy metabolism, and blood Xow in the central nervous system. FASEB J., 40, 2311±16.
SokoloV, L. (1985). Basic principles in imaging of cerebral metabolic rates. In Brain Imaging and Brain Function, ed. L. SokoloV, pp. 21±49. New York: Raven Press.
SokoloV, L., Reivich, M., Kennedy, C. et al. (1977). The [14C]deoxyglucose method for the measurement of local cerebral glucose utilization; theory, procedure, and normal values in the conscious and anesthetized albino rat. J. Neurochem., 28, 897±916.
Sorenson, J. A. and Phelps, M. E. (1987). Physics in Nuclear Medicine. Orlando, FL: Grune and Stratton.
Spence, A. M., Graham, M. M., Muzi, M. et al. (1990). Deoxyglucose lumped constant estimated in a transplanted rat astrocytic glioma by the hexose utilization index. J. Cereb. Blood Flow Metab., 10, 190±8.
Spinks, T. J., Jones, T., Bailey, D. L. et al. (1992). Physical performance of a positron tomograph for brain imaging with retractable septa. Phys. Med. Biol., 37, 1637±55.

26 P. Herscovitch and M. Ernst
Stapleton, S. J., Caldwell, C. B., Leonhardt, C. L., Ehrlich, L. E., Black, S. E. and YaVe, M. J. (1994). Determination of thresholds for detection of cerebellar blood Xow deWcits in brain SPECT images. J. Nucl. Med., 35, 1547±55.
Strother, S. C., Kanno, I. and Rottenberg, D. A. (1995). Commentary and opinion. 1. Principal component analysis, variance partitioning, and functional connectivity. J. Cereb. Blood Flow Metab.,
15, 353±60.
Subramanyam, R., Alpert, N. M., Hoop, B., Jr, Brownell, G. L. and Taveras, J. M. (1978). A model for regional cerebral oxygen distribution during continuous inhalation of 15O2, C15O, and C15O2. J. Nucl. Med., 19, 13±53.
Ter-Pogossian, M. M. (1992). The origins of positron emission tomography. Semin. Nucl. Med., 22, 140±9.
Ter-Pogossian, M. M., Phelps, M. E., HoVman, E. J. and Mullani, N. A. (1975). A positron-emission transaxial tomograph for nuclear imaging (PETT). Radiology, 114, 89±98.
Toga, A. W. and Collins, R. C. (1981). Metabolic response of optic centers to visual stimuli in the albino rat: anatomical and physiological considerations. J. Comp. Neurol., 199, 443±64.
Tyler, J. L., Strother, S. C., Zatorre, R. J. et al. (1988). Stability of regional cerebral glucose metabolism in the normal brain measured by positron emission tomography. J. Nucl. Med., 29, 631±42. van Bogeart, P., Wikler, D., Damhaut, P., Szliwowska, H. B. and Goldman, S. (1998). Regional changes in glucose metabolism during brain development from the age of 6 years. Neuroimage,
8, 62±8.
van Heertum, R. L., Miller, S. H. and Mosesson, R. E. (1993). SPECT brain imaging in neurologic disease. Radiol. Clin. North Am., 31, 881±907.
Veatch, R. M. (1982). The ethics of research involving radiation.
IRB: Rev. Hum. Subjects Res., 4, 3±5.
Videen, T. O., Perlmutter, J. S., Herscovitch, P. and Raichle, M. E. (1987). Brain blood volume, Xow, and oxygen utilization measured with 15O radiotracers and positron emission tomography: revised metabolic computations. J. Cereb. Blood Flow Metab., 7, 513±16.
Videen, T. O., Perlmutter, J. S., Mintun, M. A. and Raichle, M. E. (1988). Regional correction of positron emission tomography data for the eVects of cerebral atrophy. J. Cereb. Blood Flow Metab., 8, 662±70.
Volkow, N. and Fowler, J. S. (1992). Neuropsychiatric disorders: investigation of schizophrenia and substance abuse. Semin. Nucl. Med., 22, 254±67.
Volpe, J. J., Herscovitch, P., Perlman, J. M. and Raichle, M. E. (1983). Positron emission tomography in the newborn: Extensive impairment of regional cerebral blood Xow with intraventricular hemorrhage and hemorrhagic intracerebral involvement.
Pediatrics, 72, 589±601.
Wang, B., Thompson, C. J., Tremblay, H., Reutens, D., Jolly, D. and Meyer, E. (1998). Measurement of the evoked vascular response (EVR) to cerebral activation due to vibro-tactile stimulation using gated C-11-CO PET acquisition. IEEE Trans. Nucl. Sci., 45, 1111±16.
Wang, G.-J., Volkow, N. D., Wolf, A. P., Brodie, J. D. and Hitzeman, R. J. (1994). Intersubject variability of brain glucose metabolic measurements in young normal males. J. Nucl. Med., 35, 1457±66.
Warner, D. S., Kassell, N. F. and Boarini, D. J. (1987). Microsphere cerebral blood Xow determination. In Cerebral Blood Flow: Physiologic and Clinical Aspects, ed. J. H. Wood, pp. 288±98. New York: McGraw-Hill.
Weinberg, I. N., Huang, S. C., HoVman, E. J. et al. (1988). Validation of PET-acquired input functions for cardiac studies. J. Nucl. Med., 29, 241±7.
Wienhard, K., Pawlik, G., Herholz, K., Wagner, R. and Heiss, W.-D. (1985). Estimation of local cerebral glucose utilization by positron emission tomography of [18F]2-Xuoro-2-deoxy-D- glucose: a critical appraisal of optimization procedures. J. Cereb. Blood Flow Metab., 5, 115±25.
Wienhard, K., Dahlbom, M., Eriksson, L. et al. (1994). The ECAT EXACT HR: performance of a new high resolution positron scanner. J. Comput. Assist. Tomogr., 18, 110±18.
Wilson, J. T. L. and Wyper, D. (1992). Neuroimaging and neuropsychological functioning following closed head injury: CT, MRI, and SPECT. J. Head Trauma Rehab., 7, 29±39.
Wilson, M. W. and Mountz, J. M. (1989). A reference system for neuroanatomical localization on functional reconstructed cerebral images. J. Comput. Assist. Tomogr., 13, 174±8.
Wolf, A. P. and Schlyer, D. J. (1993). Accelerators for positron emission tomography. In Nuclear Imaging in Drug Discovery, Development, and Approval, ed. D. H. Burns, R. E. Gibson, R. F. Dannals and P. K. S. Siegl, pp. 33±54. Boston: Birkhäuser.
Woods, R. P., Mazziotta, J. C. and Cherry, S. R. (1993). MRI±PET registration with automated algorithm. J. Comput. Assist. Tomogr., 17, 536±46.
Wyper, D. J. (1993). Functional neuroimaging with single photon emission computed tomography (SPECT). Cerebrovasc. Brain Metabo. Rev., 5, 199±217.
Yarowsky, P. J. and Ingvar, D. H. (1981). Neuronal activity and energy metabolism. Fed. Proc., 40, 2353±62.
Yarowsky, P., Kadekaro, M. and SokoloV, L. (1983). Frequencydependent activation of glucose utilization in the superior cervical ganglion by electrical stimulation of cervical sympathetic trunk. Proc. Nat. Acad. Sci. USA, 80, 4179±83.
Zametkin, A. J., Liebenauer, L. L., Fitzgerald, G. A. et al. (1993). Brain metabolism in teenagers with attention deWcit hyperactivity disorder. Arch. Gen. Psychiatry, 50, 333±40.

2
Modeling of receptor images in PET and SPECT
Evan D. Morris, Raymond F. Muzic Jr, Bradley C. Christian, Christopher J. Endres, and Ronald E. Fisher
Introduction
Positron emission tomography (PET) and single photon emission computed tomography (SPECT) are the only noninvasive imaging modalities, currently, that can be used to image speciWc receptor molecules and to quantify their kinetics. The speciWcity of these methods results from the use of radiolabeled ligands that are themselves speciWc for a receptor or transporter molecule. In PET/SPECT studies, the images are a composite of various signals only one of which relates to ligand bound to the receptor site of interest. The component of the image that is from receptor binding is isolated using a mathematical model relating the dynamics of the ligand to the resultant PET image. The mathematical model typically derives from the three-compartment model in which a directly measured blood curve serves as the model's input function. (There are modiWcations of the model (reference region models) that do not use an independently measured blood curve as input. These models are addressed later in this chapter.) The model contains a number of parameters that are taken to be constants and reXective of inherent kinetic properties of the system and the particular ligand. By formally comparing the output of the model to the experimentally obtained PET or SPECT data, we can estimate values for the kinetic parameters and thus extract information on binding, or any hypothesized process, as distinct from all other processes contributing to the PET signal. In general, the information content of the PET or SPECT data is inadequate to support models of great sophistication, and so adoption of a particular model and the interpretation of its parameters comes with associated assumptions and conditions that must be satisWed.
Kinetic models
The basic compartmental model
The simplest of compartmental models applied to receptor±ligand studies postulates two tissue compartments. These two tissue compartments along with a plasma compartment are arranged in series. (Strictly speaking, the plasma is not a compartment of the model. The concentration of tracer in the plasma is measured independently and applied to the tissue model as a known input function.) The tracer is delivered, typically by intravenous injection, into the plasma and it traverses the ªfreeº compartment on its way to interacting with the receptor. If these three compartments or states of the radioligand are inadequate to describe the data, sometimes a third tissue compartment is introduced (Fig. 2.1) that is termed the nonspeciWcally bound compartment. The bound and nonspeciWc compartments are distinguished as follows. SpeciWcally bound ligand, unlike nonspeciWcally bound ligand is both saturable and displaceable by nonradioactive molecules of the same tracer. The rate of change in radioactivity concentration in each tissue compartment in Fig. 2.1 is given by the ordinary diVerential equations that describe the Xux of ligand into the respective compartments:
dF |
5 K |
P2 (k 1 k )F1 k |
B2 lF |
(2.1) |
||||
|
||||||||
dt |
1 |
2 |
3 |
4 |
|
|
||
|
|
|
|
|
|
|
||
dB |
5 k3F2 k4B2 lB |
|
|
(2.2) |
||||
dt |
|
|
||||||
|
|
|
|
|
|
|
||
dN |
5 k |
F2 k N2 lN |
|
|
(2.3) |
|||
|
|
|
|
|||||
dt |
5 |
6 |
|
|
|
|
||
|
|
|
|
|
|
|
where F, B, and N are the time-varying concentrations of tracer in the free, bound, and nonspeciWc tissue compartments, respectively; that is, F5 F(t), B5 B(t), and N5 N(t). The constants K1, k2 . . . k6 are the apparent Wrst-order rate
27
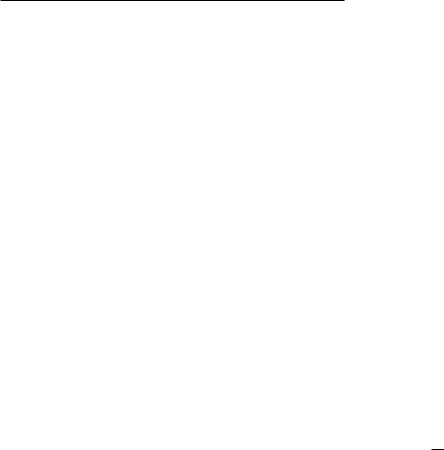
28 E. D. Morris et al.
Fig. 2.1. General three-compartment model. Radioactivity in the plasma, free, bound (and nonspeciWc) compartments sums to give the radioactivity in the PET image. The model parameters K1
. . . k6 reXect exchange rates for labeled ligand moving between various compartments.
constants relating the transfer of ligand between the various compartments. These constants are the model parameters to be estimated from experimental data. The parameters k3 and k4 are apparent Wrst-order rate constants that characterize the binding and dissociation of the ligand to and from the receptor, respectively. The rate constant l, radioactive decay, refers to the rate of decay of the isotope labeling the ligand. The (measured) concentration of radioactive ligand in the plasma P refers only to the concentration of native ligand molecules and not to any radioactive metabolites. The preceding model, in a slightly diVerent form, was introduced into the PET literature by Mintun et al. in 1984.
One of the innovations of the original Mintun formulation was to ªcollapseº the nonspeciWc compartment into the free compartment. Mathematically, this can be justiWed if the rate constants for nonspeciWc binding, k5 and k6, are fast relative to the other rate constants of the system. In this case, ligand in the nonspeciWc compartment is deWned as being ªin equilibriumº with the free compartment. If this condition is satisWed, then at any time a Wxed fraction of the free plus nonspeciWc compartment is the eVective free pool available for receptor binding. This fraction, f2 5 k6/(k5 1 k6)), may not be strictly identiWable (see below) from a receptor-rich region alone, although it may be possible to estimate it from a receptor-free region where only two other parameters (K1 and k2) are needed to describe the data.
Output equation
The measured PET data comprise average concentrations over discrete frame times. Once the model has been solved for the instantaneous activity in each compartment (F, B, and N ), these concentration curves are summed and inte-
Fig. 2.2. Simulation of [11C]-raclopride dynamics in brain. Equation (2.4) generates the PET activity (h), which is equal to the sum of the activity in the free (n) and bound (e) compartments, respectively. The free and bound compartments correspond to the model variables, F(t) and B(t), respectively. Parameters are based on Farde et al. (1989). Data are not decay corrected.
grated to generate the measured PET activity over each acquisition time frame, [ti, ti11], as follows:
ti11 |
|
PET[ti,ti11]5 %1ti E (+.V1 +f F1 +bB1 +nN)dt |
(2.4) |
ti
The weights +i that premultiply each compartment concentration in Eq. (2.4) are the respective volume fractions and V is whole blood activity. Usually the vascular volume fraction +v is small, and the tissue volume fractions are set at unity or (1-+v). We can use numerical methods to solve Eqs. (2.1±2.3) over time. A simulation of the dynamics in the free and bound compartments following bolus injection of the dopamine D2 ligand, [11C]-raclopride, is shown in Fig. 2.2. The simulation is based on published parameter estimates (Farde et al., 1989) for [11C]-raclo- pride, in vivo. As in this Wgure, nonspeciWc binding is often assumed to be negligible for raclopride.
Measuring the blood curve
The input, or driving function, P represents the amount of tracer ligand that is presented over time to the tissue of interest (i.e., the brain). It is often measured via the radioactivity counted in blood plasma samples drawn from the radial artery. The model given in Eqs. (2.1±2.4) and shown in Fig. 2.1 deals only with the behavior of a unique tracer molecule, such as [11C]-raclopride. The rate constants pertain, speciWcally, to the deWned molecule. Therefore, if metabolism of the radioligand in the periphery leads to labeled metabolites in the plasma, we must invoke at least
Modeling of receptor images in PET/SPECT |
29 |
|
|
one correction and one assumption. First, the nonnative species must be removed either physically from the blood samples or mathematically from the resulting radioactivity counts. Second, it must be assumed that the labeled metabolite(s) does not cross the blood±brain barrier. Consequently, one consideration for choosing between candidate tracers may be the generation, or not, of labeled metabolites. In some cases, the production of labeled metabolites may be inevitable. In the case of [18F]- Xuorodopa, complicated models have been introduced to account for metabolite species and their contributions to the PET signal (Huang et al., 1991).
Modeling saturability
To this point, the model does not contain a term that reXects the saturable nature of the bound-ligand compartment. That is, there is no explicit upper limit imposed on the size of the bound ligand B. And yet, a unique characteristic of receptor±ligand interactions is that, like enzyme±substrate reactions, they are saturable. The inclusion of an explicit receptor density term, B9max, requires an elaboration of Eqs. (2.1) and (2.2).We expand k3 from equations (2.1±2.3) as kon(B9max2B/SA):
dF |
5 K1P 2 (k2 1 k5)F 1 k6N 2 kon(B9max 2 B/SA)F 1 koff |
B 2 lF |
|
dt |
|
||
|
|
|
(2.5) |
dB |
5 kon(B9max 2 B/SA)F 2 koff B 2 lB |
(2.6) |
|
dt |
|||
dN |
5 k5F 2 k6N 2 lN |
(2.7) |
|
|
|
||
dt |
|
|
where koV is equivalent to k4, above; SA is the speciWc activity, which declines in time according to the radioactive decay rate l; kon is the rate constant for a bimolecular association of free ligand and available receptors. Therefore, the binding rate is equal to the product of the concentrations of free ligand, F, available receptor sites,
(B9max 2 B/SA), and rate constant, kon. At the time of injection (t5 0), the value of B is zero; therefore B9max represents
the concentration of available receptors in the absence of exogenous ligand. As additional receptors become bound to ligand molecules, the availability of free receptors drops and saturation is approached asymptotically. Since availability can be aVected by either labeled or unlabeled ligand, normalization by SA converts B to concentration of total ligand. This form of the receptor±ligand model, which was Wrst proposed explicitly by Huang and colleagues (Huang et al., 1989, 1986; Bahn et al., 1989) is analogous to the bimolecular interaction between substrate, and available binding sites on an enzyme.
Parameter identiWability: opting for binding potential or other compound parameters
Despite the inclusion of an explicit B9max term in the model representing the baseline level of available receptor sites, this value may not be discernible from the acquired data. In other words, it would not be estimated reliably. Consider that for a single bolus injection of suYciently high SA ligand, the bimolecular binding term in Eqs. (2.5) and (2.6)
reduces to kon(B9max)F; that is, B/SA5 0. Since kon and B9max always appear as a product, never separately, they are said
not to be identiWable and the model defaults to Eqs. (2.1±2.3). Attempting to estimate both kon and B9max in this case would be akin to trying to estimate m and n from data with the overparameterized model of a line: y5 m*n*x1 b. Mintun and co-authors made this point in their 1984 paper saying that kon and B9max are not separable in a single bolus experiment under tracer (i.e., high SA) conditions. For that reason, they chose to estimate the compound term B9max/KD, which they referred to as ªbinding potentialº. Koeppe et al. (1991) recommend further model simpliW- cations when it is not possible even to identify B9max/KD, explicitly. Sometimes the data from a receptor±ligand study can be Wtted to a two-compartment model (i.e., plasma and free) and inclusion of an explicit bound compartment is not justiWed. This will be true if the binding and dissociation rate constants for the ligand, k3 and k4, are much faster than the inXux and eZux constants, K1 and k2. When this is true, the estimated k2 will represent an apparent eZux rate constant, [k02 5 k2/(11
k5/k6 1 B9max/KD)]. This parameter may still reXect the binding potential, as indicated by the B9max/KD term, and
may be the only reliable index of binding that can be estimated. Koeppe and colleagues have done much work to characterize the kinetics of Xumazenil and other muscarinic cholinergic ligands. They point out that quantiWcation is diYcult if the dissociation rate constant koV is slow and cannot be reliably distinguished from zero (as is the case with these ligands). Conversely, quantiW- cation of binding rate constants of these ligands is diYcult and highly sensitive to changes in blood Xow when binding is very rapid relative to the rates of transport across the blood±brain barrier (i.e., K1 and k2) (Zubieta et al., 1998).
There are many instances in research when binding potential (BP) is a satisfactory indicator of (available) receptor level. In these cases, BP, reported in table form by brain region or as a parametric map, is a reasonable endpoint for a functional imaging study. A number of easy and robust methods for calculating this parameter are discussed below. However, implicit in the adoption of BP as a surrogate B9max is the assumption that the aYnity KD of the

30 E. D. Morris et al.
Fig. 2.3. Dynamic PET activity from a region drawn on the striatum of a Rhesus monkey. The three-injection protocol used is described in Morris et al. (1996a). Injections of [11C]-CFT were made at 0, 30, and 90min (indicated by arrows). Second injection was of low speciWc activity, causing net displacement of labeled ligand from the receptor by unlabeled ligand. Notice the diVerence in responses after the
Wrst and second high speciWc activity injections because of the presence of residual cold ligand on-board. Data are not decay corrected.
ligand for the receptor is constant across subjects or treatments. For those cases where changes in aYnity cannot be excluded or where some other mechanistic question is at stake, more than a single bolus injection is indicated. Delforge et al. (1989, 1990) proposed an experimental protocol involving three sequential bolus injections of varying speciWc activities. At least one of those injections also contained a nontrace amount of cold (i.e., unlabeled) ligand. In such a protocol, the number of available receptors is signiWcantly perturbed and it is this process of modulating availability over a broad range of values that serves to de-correlate kon from B9max. Delforge and co-authors showed through experiments and simulations that it was possible to estimate unambiguously seven model parameters (including kon, B9max and koV) using a three-injec- tion protocol. In this way, the individual parameters are said to be identiWable. An example of the data from a multiple injection protocol, in which [11C]-CFT (a dopamine transporter ligand) was administered to a rhesus monkey, is given in Fig. 2.3. The authors of the study (Morris et al., 1996a) were able to estimate Wve parameters simultaneously (nonspeciWc binding parameters k5 and k6 were Wxed based on cerebellum data). In designing experiments, one must beware that protocols that rely on nontrace doses of ligand may not be feasible in psychiatric patients ± particularly children ± because the large mass doses of ligand are likely to have pharmacologic eVects.
Modeling multiple-injection data: two parallel models
To analyze data from their multiple-injection experiments, Delforge and colleagues proposed a further modiWcation of the three compartment model. Because these protocols depend on at least one nontrace injection of ligand (nontrace meaning an amount suYcient to have visible biological or pharmacologic eVects), the researchers proposed parallel models for the labeled and unlabeled species. The model can be described as follows:
dF j
dt 5 K1Pj2(k21k5)Fj1k6Nj2(kon/VR)(B9max2Bh2Bc)Fj
|
|
1k |
oV |
Bj2ljFj |
|
|
|
(2.8) |
|||
|
|
|
|
|
|
|
|
|
|
|
|
dB j |
|
|
|
|
|
|
2Bh 2Bc)Fj2k |
|
Bj2ljBj |
|
|
|
5 (k /V |
)(B9 |
max |
oV |
(2.9) |
||||||
|
|||||||||||
dt |
on |
R |
|
|
|
|
|
||||
|
|
|
|
|
|
|
|
|
|
||
dN j |
5 (k Fj2k |
Nj2ljN j |
|
|
(2.10) |
||||||
|
|
|
|
||||||||
dt |
5 |
|
6 |
|
|
|
|
|
|
||
|
|
|
|
|
|
|
|
|
|
where the superscript j refers to either hot (h) or cold (c) ligand; lj is the radioactive decay constant for the isotope when j refers to the hot ligand and is 0 when j refers to cold (i.e., there is no radiodecay of cold ligand).
The Delforge nomenclature employs the compound term kon/VR as the apparent association rate constant, where VR is the volume of reaction of the receptor but kon/VR is treated as one indivisible parameter. If the microenvironment surrounding the receptor is unusually inaccessible to the ligand, the apparent binding rate will diVer from the true binding rate (see Delforge et al. (1996) for a thorough discussion of the reaction-volume concept).
Modeling of receptor images in PET/SPECT |
31 |
|
|
The number of estimable parameters in the model is seven. No new parameters have been added to the model given earlier (Eqs. (2.4±2.6)) because the hot and cold ligands are identical, biologically.
There is an additional experimental burden in performing multiple-injection experiments. Two plasma input functions must be measured. One, Ph, is the standard curve of radioactivity in the plasma, which has been mentioned previously. The other Pc is the concentration (in picamoles per milliliter) of cold ligand in the plasma. Since this is not readily measurable during the experiment, it must be ªreconstructedº from the available plasma radioactivity measurements and knowledge of the speciWc activities of each injection. (See the appendix of Morris et al., 1996a.) Despite its greater complexity, the Delforge approach maximizes the number of model parameters that can be properly identiWed (Morris et al., 1996b). This complexity could be justiWed in the preliminary development and exploration of a new ligand. The result of such a multiple-injection study would then be used to develop a simpler clinical protocol.
Nonconstant coeYcients: neurotransmitter changes
Each of the foregoing models, regardless of complexity, is founded on some common assumptions. One is that the model parameters are constant over the duration of the experiment. This is a reasonable assumption if the system itself is at steady state. Whereas [11C]-raclopride levels may rise and fall as it is taken up and eliminated from the tissue following a bolus injection, dopamine levels remain unchanged over the timescale of interest. The rate constants that are determined by the overall state of the system remain unchanged. Anything that violates these assumptions may invalidate the use of the constantcoeYcient models given earlier and must be investigated. For example, the consequences of unwanted changes in blood Xow and their impact on estimation of raclopride binding have been investigated using both simulations (Frost et al., 1989; Laruelle et al., 1994; Logan et al., 1994) and an experimental perturbation, hyperventilation (Logan et al., 1994). In 1991, Logan and colleagues explored the consequences of changes in dopamine on the measurement of binding parameters of a D2 receptor ligand, methylspiroperidol.
Of late, there has been growing interest in detecting and quantifying transient changes in neurotransmitter concentrations, which may be useful for understanding the etiology of neuropsychiatric diseases (see Laruelle et al. (1996) and Breier et al. (1997) for evidence that schizophrenia is associated with abnormal amphetamine-
induced dopamine release). The potential for such a measurement exists because the endogenous ligand (e.g., dopamine) competes with the exogenous ligand for binding to available receptor sites. Any alteration in the steady-state level of this everpresent competition could alter the PET signal. To model a system in which the neurotransmitter concentration is not constant, we must again extend the models developed above. Just as the Delforge model (Eqs. (2.8±2.10)) extended its antecedents by adding terms for labeled and unlabeled exogenous ligand species, the changing-neurotransmit- ter model must add terms for the endogenous ligand species. Morris and co-workers (1995) introduced a diVerential equation into the receptor±ligand model to describe the binding and release of endogenous species from the receptor:
dBen |
5 (ken |
/Ven)(B |
2Bh 2Bc2Ben)Fen2ken |
Ben |
(2.11) |
|
|||||
dt |
on |
R max |
oV |
|
|
|
|
|
|
|
where the superscript en refers to the endogenous species. Note that Bmax is the concentration of all the receptor sites (available and not) and the bimolecular binding terms for the other species (hot and cold tracer ligand) must be modiWed accordingly. The term Fen is the time-varying concentration of endogenous chemical available for binding to the receptor and (konen/VenR ) is the apparent binding rate constant for the endogenous ligand. Equation (2.11) can be solved simultaneously with Eqs. (2.8±2.10). A similar approach was taken by Endres and colleagues (1997) who retained the format of Eqs. (2.1±2.3) but intro-
duced a time-varying binding rate parameter k3 5 k3Bfree (t) where Bfree (t) is just Bmax 2 Bh 2 Bc 2 Ben. Here Bmax is still constant, but Bh, Bc and Ben can all vary with time. This
ªenhanced modelº (as described by Eqs. (2.8±2.11)) is shown in Fig. 2.4.
There have been two experimental approaches to the problem of quantifying neurotransmitter changes. The Wrst, is a natural extension of the studies already mentioned. It is based on the comparison of PET signals from two bolus injections of radiotracer. One bolus study is performed while neurotransmitter is at steady state; a second bolus study is carried out while endogenous neurotransmitter is undergoing an induced change. An alternative experimental approach also involves a perturbation of the neurotransmitter but it is done only after the radiotracer has been brought to a steady state in the tissue by the combination of bolus plus infusion administrations. An approach intermediate between these has been proposed by Friston et al. (1997) but is not discussed further here. In this approach a single bolus study would be performed and any statistically signi®cant deviation in time from the

32 E. D. Morris et al.
Fig. 2.4. Schematic diagram corresponding to the model described in Eqs. (2.8±2.11). Abbreviations and terms are described in the text.
predicted dynamic curve would be a measure of ªactivationº, in eVect detecting a change in k2. A pharmacologic challenge was used as a test case.
The steady-state technique
The bolus-plus-infusion method was developed for receptor±ligand characterization studies by Carson and colleagues at the National Institutes of Health (Carson et al., 1993). The beauty of this method is that by properly combining a bolus with a constant infusion of radiotracer, the radiotracer level in the brain can be brought to a constant level in a minimum of time. With ligand levels in the tissue no longer varying in time, the derivatives on the lefthand sides of Eqs. (2.1±2.3) disappear and the models reduce to a set of algebraic equations. In fact, no modeling is required. For many ligands, this technique is ideal for
reliably estimating distribution volume (which is a linear function of BP). The steady-state method has been used quite extensively in SPECT studies (Laruelle et al., 1994), which, because of long-lived isotopes, can be applied to data acquired the day following a bolus injection (no infusion needed). The bolus-plus-infusion method is easily extended to neurotransmitter-change experiments by perturbing the endogenous neurotransmitter pharmacologically once the steady-state level of radiotracer has been achieved. The measured parameter that has been used to indicate neurotransmitter alteration is the change in binding potential, %BP. Fig. 2.5 shows a change in the steady-state level of raclopride activity in the striatum of a monkey before and after amphetamine perturbation. The activity in the cerebellum (devoid of D2 receptors) is unaVected (Endres et al., 1997).

Fig. 2.5. The eVect of amphetamine (0.4mg/kg) on raclopride levels in the brain of monkeys using the bolus-plus-infusion method for detecting a change in neurotransmitter level. The [11C]-raclopride signal in the striatum is depressed following heightened competition between raclopride and dopamine for binding at D2 receptors. The activity in the cerebellum, which has no D2 receptors, is unchanged. The dotted continuation of the top curve shows the model-predicted continuation of the curve in the absence of an amphetamine injection. Data are decay corrected. (Data from Endres et al., 1997.)
Experimental considerations
Whether one chooses the two-bolus or the constant-infu- sion approach, there are two important questions to be answered. Can the change in PET signal be reliably detected given the inherent variability in the method? Can the apparent change in some parameter of interest be unambiguously linked to changes in neurotransmitter, given the inherent variability and covariability of the parameters? With regard to the former, Endres et al. (1997) used simulations to show that with the bolus-plus-infusion technique, %BP is proportional to the integral of the endogenous neurotransmitter curve (i.e., the additional amount of neurotransmitter, released transiently, above the steady state level). Morris, Fisher and colleagues at Massachusetts General Hospital (Fisher et al., 1995; Morris et al., 1995) showed that with the two-bolus method, the sum of squares diVerence (&2) between the two dynamic PET curves (with and without perturbation) increases with duration of perturbation. Importantly, the eVect of transient neurotransmitter release is distinct from a transient increase in blood Xow and the precise timing of the perturbation relative to the tracer bolus can greatly aVect the magnitude of the signal change. Endres and Carson (1998) provided a mathematic formalism for understanding the importance of perturbation timing and its relation to the kinetics of the labeled ligand. The implications of these techniques and some recent applications are examined at the end of this chapter.
Modeling of receptor images in PET/SPECT |
33 |
|
|
Limitations to absolute quantiWcation
To this point, we have examined models that describe the concentration of a tracer molecule in various states, or compartments, in the tissue. We have asserted that by summing the concentrations of radioactive tracer in each state postulated by the model, we can predict the measured PET signal, and by comparing the prediction with data we can quantify some useful model parameters. Sometimes, however, for reasons related to the scanner, the measured signal in a region of interest does not reXect the true concentration of tracer in a given tissue. Therefore, before binding or other parameters of interest can be estimated, the physics of the measurements must be considered.
Resolution eVects
When the structure of interest (e.g., striatum) is small in comparison to the resolution characteristics of the scanner, the radioactivity measured in that structure is a biased estimate of its true radioactivity concentration. This is often referred to generically as the partial volume eVect. Figure 2.6 illustrates this point. The radioactivity in the striatum is artifactually reduced from its true value, while it is artifactually increased in adjacent brain regions. How signiWcant is this bias or error in radioactivity measurement? As discussed in Chapter 1, the resolution of PET or SPECT scanners aVects the accuracy of the images obtained. This resolution is commonly expressed in terms of the full-width-at-half-maximum (FWHM) (Fig. 2.7). A widely quoted rule of thumb is that the radioactivity in a sphere that is embedded in a nonradioactive background is accurate to within 2% if the sphere's diameter is greater than 2.7 times the FWHM (Kessler et al., 1984). This may be overly optimistic. Chen et al. (1998) observed underestimation of 10 to 20% of true radioactivity for a sphere that was more than three times the FWHM of their modern scanner (FWHM5 4.5mm, in-plane). Regardless of the speciWc numbers, the bias is inevitable and will probably be signiWcant in measuring many of the smaller brain structures. The best partial resolution reported to date is approximately 3mm with PET (Valk et al., 1990). The spatial resolution of SPECT is much worse (typically $10 mm, in-plane).
The important question for the design of quantitative PET/SPECT studies is how will the error in measured radioactivity aVect measures of receptor function that are obtained using any of the modeling analyses presented above? The answer, in part, depends on the particular model used to analyze the data and the timing and amount