
Kluwer - Handbook of Biomedical Image Analysis Vol
.2.pdf222 |
Kallergi, Hersh, and Manohar |
[11]Haaga, J. R., Alfide, R. J., Zelch, M. G., Meany, T. F., Boller, M., Gonzalez, L., and Jelden, G. L., Computed tomography of the pancreas, Radiology, Vol. 120, pp. 589–595, 1976.
[12]Haaga, J. R., Alfide, R. J., Harvilla, T. R., Tubbs, R., Gonzalez, L., Meany,
T.F., and Corsi, M. A., Definitive role of CT scanning of the pancreas: The second year’s experience, Radiology, Vol. 124, pp. 723–730, 1977.
[13]Sheth, S., Hruban, R. K., and Fishman, E. K., Helical CT of islet cell tumors of the pancreas: Typical and atypical manifestations, AJR, Vol. 179, pp. 725–730, 2002.
[14]Horton, K. M. and Fishman, E. K., Adenocarcinoma of the pancreas: CT imaging, Radiol. Clin. North Am., Vol. 40, pp. 1263–1272, 2002.
[15]Horton, K. M., Multidetector CT and three-dimensional imaging of the pancreas: state of the art, J. Gastrointest. Surg., Vol. 6, pp. 126–128, 2002.
[16]Winston, C. B., Mitchell, D. G., Outwater, E. K., and Ehrlich, S. M., Pancreatic signal intensity on T1-weighted fat saturation MR images: Clinical correlation, J. Magn. Reson. Imaging, Vol. 5, pp. 267–271, 1995.
[17]Ragozzino, A. and Scaglione, M., Pancreatic head mass: What can be done? Diagnosis: Magnetic resonance imaging, J. Pancreas, Vol. 1, pp. 100–107, 2000.
[18]Barish, M. A., Yucel, E. K., and Ferrucci, J. T., Magnetic resonance cholangiopancreatography, NEJM, Vol. 341, pp. 258–264, 1999.
[19]Fulcher, A. S. and Turner, M. A., MR pancreatography: A useful tool for evaluating pancreatic disorders, Radiographics, Vol. 19, pp. 5–24, 1999.
[20]Adamek, H. E., Albert, J., Breer, H., Weitz, M., Schilling, D., and Riemann,
J.F., Pancreatic cancer detection with magnetic resonance cholangiopancreatography and endoscopic retrograde cholangiopancreatography: a prospective controlled study, Lancet, Vol. 356, pp. 190–193, 2000.
Automatic Segmentation of Pancreatic Tumors in Computed Tomography |
223 |
[21]Mertz, H. R., Sechopoulos, P., Delbeke, D., and Leach, S. D., EUS, PET, and CT scanning for evaluation of pancreatic adenocarcinoma, Gastrointest. Endosc., Vol. 52, pp. 367–371, 2000.
[22]Wiersema, M. J., Accuracy of endoscopic ultrasound in diagnosing and staging pancreatic carcinoma, Pancreatology, Vol. 1, pp. 625–632, 2001.
[23]Kalra, M. K., Maher, M. M., Boland, G. W., Saini, S., and Fischman,
A.J., Correlation of positron emission tomography and CT in evaluating pancreatic tumors: Technical and clinical implications, AJR, Vol. 181, No. 2, pp. 387–393, 2003.
[24]Koyama, K., Okamura, T., Kawabe, J., Nakata, B., Hirakawa-Chung, K.
Y.S., Ochi, H., and Yamada, R., Diagnostic usefulness of FDG PET for pancreatic mass lesions, Ann. Nuclear Med., Vol. 15, No. 3, pp. 217–224, 2001.
[25]Dupuy, D. E., Costello, P., and Ecker, C. P., Spiral CT of the pancreas, Radiology, Vol. 183, pp. 815–818, 1992.
[26]DiChiro, G. and Brooks, R. A., The 1979 Nobel prize in physiology and medicine, Science, Vol. 206, No. 30, pp. 1060–1062, 1979.
[27]Kalender, W. A. and Polacin, A., Physical performance characteristics of spiral CT scanning, Med. Phys., Vol. 18, No. 5, pp. 910–915, 1991.
[28]Boone, J. M., Computed tomography: Technology update on multiple detector array scanners and PACS considerations, In: Practical Digital Imaging and PACS, Seibert, J. A., Filipow L. J., and Andriole, K. P., eds., AAPM Medical Physics Monograph No. 25, Medical Physics Publishing, Madison, WI, pp. 37–65, 1999.
[29]Swindell, W. and Webb, S., X-ray transmission computed tomography, In: The Physics of Medical Imaging, Webb, S., ed., Adam Hilger, Bristol, pp. 98–127, 1988.
[30]McCollough, C. H. and Zink, F. E., Performance evaluation of a multislice CT system, Med. Phys., Vol. 26, No. 11, pp. 2223–2230, 1999.
224 |
Kallergi, Hersh, and Manohar |
[31]Sheedy, P. F., II., Stephens, D. H., Hattery, R. R., MacCarty, R. L., and Williamson, B., Jr., Computer tomography of the pancreas, Radiol. Clin. North Am., Vol. 15, No. 3, pp. 349–366, 1977.
[32]Dendy, P. P. and Heaton, B., Physics for Diagnostic Radiolog, 2nd ed., Medical Science Series, Institute of Physics Publishing, Bristol, 1999.
[33]Remer, E. M. and Baker, M. E., Imaging of chronic pancreatitis, Radiol. Clin. North Am., Vol. 40, pp. 1229–1242, 2002.
[34]Love, L., (guest ed.), Symposium on abdominal imaging, Radiol. Clin. North Am., Vol. 17, No. 1, 1979.
[35]Frank Miller, H., (guest ed.), Radiology of the pancreas, gallbladder, and biliary tract, Radiol. Clin. North Am., Vol. 40, No. 6, 2002.
[36]Sheth, S. and Fishman, E. K., Imaging of uncommon tumors of the pancreas, Radiol. Clin. North Am., Vol. 40, pp. 1273–1287, 2002.
[37]Stanley, R. J. and Semelka, R. C., Pancreas, In: Computed Body Tomography with MRI Correlation, Lee, J. K. T., Sagel, S. S., Stanley, R. J., and Heiken, J. P., eds., Lippincott Raven, pp. 915–936, 1998.
[38]Sheedy, P. F., II, Stephens, D. H., Hattery, R. R., MacCaty, R. L., and Williamson, B., Jr., Computed Tomography of the Pancreas: Whole Body Computed Tomography, Radiol. Clin. North Am., Vol. 15, No. 3, pp. 349– 366, 1977.
[39]Masero, V., Leon-Rojas, J. M., and Moreno, J., Volume reconstruction for health care: A survey of computational methods, Ann. N Y Acad. Sci., Vol. 980, pp. 198–211, 2000.
[40]Udupa, J. K., Three-dimensional visualization and analysis methodologies: A current perspective, Radiographics, Vol. 19, No. 3, pp. 783–806, 1999.
[41]Gonzalez, R. C. and Woods, R. E., (Eds.), Digital Image Processing, 2nd edn., Computer Science Press, Prentice Hall, NJ, 2002.
[42]Kobashi, M. and Shapiro, L. G., Knowledge-based organ identification from CT images, Patt. Recogn., Vol. 28, No. 4, pp. 475–491, 1995.
Automatic Segmentation of Pancreatic Tumors in Computed Tomography |
225 |
[43]Dawant, B. M. and Zijdenbos, A. P., Image segmentation, In: Handbook of Medical Imaging, Volume 2: Medical Image Processing and Analysis, Fitzpatrick, J. M. and Sonka, M., eds., SPIE, pp. 71–127, 2000.
[44]Schiemann, T., Michael, B., Tiede, U., and Hohne, K. H., Interactive 3Dsegmentation, SPIE, Vol. 1808, pp. 376–383, 1992.
[45]Ikeda, M., Shigeki, I., Ishigaki, T., and Yamauchi, K., Evaluation of a neural network classifier for pancreatic masses based on CT findings, Comput. Med Imaging Graphics, Vol. 21, No. 3, pp. 175–183, 1997.
[46]Clarke, L. P., Velthuizen, R. P., Camacho, M. A., Heine, J. J., Vaidyanathan, M., Hall, L. O., Thatcher, R. W., and Silbiger, M. L., Review of MRI segmentation: Methods and applications, Magn. Reson. Imaging, Vol. 13, No. 3, pp. 343–368, 1995.
[47]Bensaid, A. M., Improved Fuzzy Clustering for Pattern Recognition with Applications to Image Segmentation., Ph.D. Dissertation, Department of Computer Science, University of South Florida, 1994.
[48]Bezdek, J. C., Pattern Recognition with Fuzzy Objective Function Algorithm, Plenum Press, New York, 1981.
[49]Bensaid, A. M., Bezdek, J. C., Hall, L. O., and Clarke, L. P., A partially supervised fuzzy c-means algorithm for segmentation of MR images, SPIE, Vol. 1710, pp. 522–528, 1992.
[50]Bensaid, A. M., Hall, L. O., Bezdek, J. C., Clarke, L. P., Silbiger, M. L., Arrington, J. A., and Murtagh, R. F., Validity-guided (re)clustering with application to image segmentation, IEEE Trans. Fuzzy Sys., Vol. 4, No. 2, pp. 112–123, 1996.
[51]Clark, M. C., Hall, L. O., Goldgof, D. B., Clarke, L. P., Velthuizen, R. P., and Silbiger, M. S., MRI segmentation using fuzzy clustering techniques, IEEE Eng. Med. Biol. Magazine, Vol. 13, No. 5, pp. 730–742, 1994.
[52]Clarke, L. P., Velthuizen, R. P., Phuphanich, S., Schellenberg, J. D., Arrington, J. A., and Silbiger, M. L., MRI: Stability of three supervised segmentation techniques, Magn. Reson. Imaging, Vol. 11, No. 1, pp. 95– 106, 1993.
226 |
Kallergi, Hersh, and Manohar |
[53]Vaidyanathan, M., Clarke, L. P., Velthuizen, R. P., Phuphanich, S., Bensaid, A. M., Hall, L. O., Bezdek, J. C., Greenburg, H., Trotti, A., and Silbiger, M., Comparison of supervised MRI segmentation methods for tumor volume determination during therapy, Magn. Reson. Imaging, Vol. 13, No. 5, pp. 719–728, 1995.
[54]Velthuizen, R. P., Clarke, L. P., Phuphanich, S., Hall, L. O., Bensaid, A. M., Arrington, J. A., Greenberg, H. M., and Silbiger, M. L., Unsupervised measurement of brain tumor volume on MR images, J. Magn. Reson. Imaging, Vol. 5, No., 5, pp. 594–605, 1995.
[55]Velthuizen, R. P., Hall, L. O., and Clarke, L. P., An initial investigation of feature extraction with genetic algorithms for fuzzy clustering, Biomed. Eng., Appl., Basis Commun., Vol. 8, No. 6, pp. 496–517, 1996.
[56]Velthuizen, R. P. and Gangadharan, D., Mammographic mass classification: Initial results, In: SPIE Medical Imaging Conference, San Diego, CA, February 12–18, 2000.
[57]Li, L., Zheng, Y., Kallergi, M., and Clark, R. A., Improved method for automatic identification of lung regions on chest radiographs, Acad. Radiol., Vol. 8, pp. 629–638, 2001.
[58]Kallergi, M., Carney, G., and Gaviria, J., Evaluating the performance of detection algorithms in digital mammography, Med. Phy., Vol. 26, No. 2, pp. 267–275, 1999.
[59]Kallergi, M., Clark, R. A., and Clarke, L. P., Medical image databases for CAD applications in digital mammography: Design issues, In: Medical Informatics Europe ’97, Pappas, C., Maglaveras, N., and Scherrer, J. R., eds., IOS Press, Amsterdam, pp. 601–605, 1997.
[60]Harrell, F. E., Lee, K. L., and Mark, D. B., Tutorial in biostatistics. Multivariate prognostic models: Issues in developing models, evaluating assumptions and adequacy, and measuring and reducing errors, Stat. Med., Vol. 15, pp. 361–387, 1996.
[61]Roe, C. A. and Metz, C. E., Dorfman–Berbaum–Metz method for statistical analysis of multireader, multimodality receiver operating
Automatic Segmentation of Pancreatic Tumors in Computed Tomography |
227 |
characteristic data: Validation with computer simulation, Acad. Radiol., Vol. 4, pp. 298–303, 1997.
[62]Li, L., Zheng, Y., Kallergi, M., and Clark, R. A., Improved method for automatic identification of lung regions on chest radiographs, Acad. Radiol., Vol. 8, pp. 629–638, 2001.
[63]Pavlidis, T., Algorithms for Graphics and Image Processing, Computer Science Press, Rockville, MD, 1982.
[64]Greenberg, S., Aladjem, M., Kogan, D., and Dimitrov, I., Fingerprint image enhancement using filtering techniques, In: International Conference on Pattern Recognition, Vol. 3, Barcelona, Spain, Sept. 3–8, 2000.
[65]Heine, J. J., Deans, S. R., Cullers, D. K., Stauduhar, R., and Clarke, L. P., Multiresolution statistical analysis of high resolution digital mammograms, IEEE Trans. Med. Imaging, Vol. 16, pp. 503–15, 1997.
[66]Weaver, J. B., Xu, Y. S., Healy, D. M., Jr., and Cromwell, L. D., Filtering noise from images with wavelet transforms, Magn. Reson. Med., Vol. 21, No. 2, pp. 288–295, 1991.
[67]Hall, L. O., Bensaid, A. M., Clarke, L. P., Velthuizen, R. P., Silbiger, M. L., and Bezdek, J., A Comparison of neural network and fuzzy clustering techniques in segmenting magnetic resonance images of the brain, IEEE Trans. Neural Networks, Vol. 3, No. 5, pp. 672–682, 1992.
[68]Phillips, W. E., Velthuizen, R. P., Phuphanich, S., Hall, L. O., Clarke, L. P., and Silbiger, M. L., Application of fuzzy c-means segmentation technique for tissue differentiation in MR images of a hemorrhagic glioblastoma multiforme, Magn. Reson. Imaging, Vol. 13, No. 2, pp. 277–290, 1995.
[69]Kallergi, M., Gavrielides, M. A., He, L., Berman, C. G., Kim, J. J., and Clark, R. A., A simulation model of mammographic calcifications based on the ACR BIRADS, Acad. Radiol., Vol. 5, pp. 670–679, 1998.
[70]Kallergi, M., He, L., Gavrielides, M., Heine, J. J., and Clarke, L. P., Resolution effects on the morphology of calcifications in digital mammograms, In: Proceedings of VIII Mediterranean Conference on Medical and
228 |
Kallergi, Hersh, and Manohar |
Biological Engineering and Computing, Medicon’ 98, Lemesos, Cyprus,
(June 14–17, 1998), CD-ROM, ISBN 9963-607-13-6.
[71]Zhang, Y. J., A review of recent evaluation methods for image segmentation, In: Proceedings of International Symposium on Signal Processing and its Applications, Malaysia, August 13–16, 2001.
[72]Zhang, Y. J., A survey on evaluation methods for image segmentation, Patt. Recogn., Vol. 29, No. 8, pp. 1335–1346, 1996.
[73]Gerig, G., Jomier, M., and Chakos, M., Valmet: A new validation tool for assessing and improving 3D object segmentation, MICCAI, Vol. 2208, pp. 516–528, 2001.
[74]Chalana, V. and Kim, Y., A methodology for evaluation of boundary detection algorithms on medical images, IEEE Trans. Med. Imaging, Vol. 16, No. 5, pp. 642–652, 1997.
[75]Kelemen, A., Szekely,´ G., and Gerig, G., Elastic model-based segmentation of 3-D neuroradiological data sets, IEEE Trans. Med. Imaging, Vol. 18, No. 10, pp. 828–839, 1999.
[76]Motulsky, H., Intuitive Biostatistics, Oxford University Press, USA, 1995.
[77]Mould, R. F., Introductory Medical Statistics, 3rd edn., Institute of Physics Publishing, Bristol, 1998.
[78]Metz, C. E., ROC methodology in radiologic imaging, Invest. Radiol., Vol. 21, pp. 720–733, 1986.
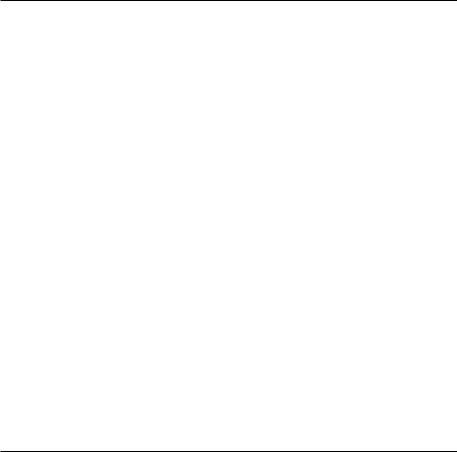
Chapter 5
Computerized Analysis and Vasodilation Parameterization in Flow-Mediated Dilation Tests from Ultrasonic Image Sequences
Alejandro F. Frangi,1,2 Martın´ Laclaustra,3 and Jian Yang1
5.1 Introduction
Assessment and characterization of endothelial function in the diagnosis of cardiovascular diseases is a current clinical research topic [1, 2]. The endothelium shows measurable responses to flow changes [3, 4], and flow-mediated dilation (FMD) may therefore be used for assessing endothelial health; B-mode ultrasonography (US) is a cheap and noninvasive way to estimate this dilation response [5]. However, complementary computerized image analysis techniques are still very desirable to give accuracy and objectivity to the measurements [1].
Several methods based on the detection of edges of the arterial wall have been proposed over the last 10 years. The first studies used a tedious manual procedure [5], which had a high interobserver variability [6]. Some interactive methods tried to reduce this variability by attracting manually drawn contours to image features, like the maximum image gradient, where the vessel wall is assumed to be located [7–9]. Some more recent efforts are focused on dynamic programming or deformable models [10–19] and on neural networks [20].
1 Computer Vision Lab, Aragon Institute of Engineering Research, University of Zaragoza,
Zaragoza, Spain
2 Department of Technology, Pompeu Fabra University, Barcelona, Spain
3 Lozano Blesa University Clinical Hospital, Aragon Institute of Health Sciences, Zaragoza,
Spain
229
230 |
Frangi, Laclaustra, and Yang |
All these methods present some common limitations. First, edge detection techniques are undermined by important error sources like speckle noise or the varying image quality typical of US sequences. Second, most methods require expert intervention to manually guide or correct the measurements thus being prone to introduce operator-dependent variability. Also, almost no method performs motion compensation to correct for patient and probe position changes. This could easily lead to measuring arterial dilation using wrong anatomical correspondences. Temporal continuity is another aspect that has not been exploited enough in previous work. Two consecutive frames have a high correlation, and only Newey and Nassiri [20] and Fan et al. [15] take advantage of this feature during edge detection. Finally, there is a general lack of large-scale validation studies in most of the techniques presented so far.
In this chapter a method is proposed that is based on a global strategy to quantify flow-mediated vasodilation. We model interframe arterial vasodilation as a superposition of a rigid motion (translation and rotation) and a scaling factor normal to the artery. Rigid motion can be interpreted as a global compensation for patient and probe movements. The scaling factor explains arterial vasodilation. The US sequence is analyzed in two phases using image registration to recover both rigid motion and vasodilation. Image registration uses normalized mutual information [21] and a multiresolution framework [22]. Temporal continuity of registration parameters along the sequence is enforced with a recursive filter. Application of constraints on the vasodilation dynamics is a natural step, since the dilation process is known to be a gradual and continuous physiological phenomenon.
Once a vasodilation curve is obtained, clinical measurementes must be extracted from it. Classically, FMD is quantified by measuring the peak vasodilation diameter relative to the basal diameter level, which are usually manually identified in the curve. Automatically extracting these two parameters is not trivial (e.g., a mere mean of several basal frames and a simple search for a maximum in the curve) given that the curve may also include artifacts. We examined the use of a robust principal component analisys to derive intuitive morphological parameters from the curves and relate them to classical FMD indexes and cardiovascular (CVD) risk factors.
The chapter is organized as follows: Section 5.2 describes the system for image acquisition and the protocol for a typical FMD study. It also describes the population used to evaluate our technique. Section 5.3 introduces the proposed method to assess FMD. The validation of the technique is reported
Computerized Analysis and Vasodilation Parameterization of FMD |
231 |
in section 5.4. In section 5.5 a novel parameterization of the vasodilation curve is introduced, and correlation analyses are presented that relate these new parameters to CVD risk factors and classical FMD parameters. In section 5.6 the results are discussed and some concluding remarks are made in section 5.7.
5.2 Materials
5.2.1 Subjects
A total of 195 sequences of varying image quality were studied, corresponding to 195 male volunteers of the Spanish Army (age range, 34–36 years). This sample is part of the AGEMZA Study, a national cohort study of cardiovascular risk factors in young adults and includes subjects with a wide range of clinical characteristics (body weight: 62.3–111.8 Kg; body mass index: 20.59–35.36 Kg/m2; hypertension: 9%; hypercholesterolemia: 20%; smokers: 24%).
5.2.2 Image Acquisition
Image acquisition was carried out at the Lozano Blesa University Hospital (Zaragoza, Spain). The echographic probe was positioned onto the arm of the patient lying supine on a bed. A silicon gel was used as impedance adapter for better ultrasound wave transmission. The probe, once the correct orientation angle was found, was fixed with a probe holder to the table where the patient’s arm lies (Fig. 5.1). Telediastolic images were captured and hold, coincident with the peak of the R wave of the electrocardiogram. A SONOS 4500 (Agilent Technologies, Andover, MA, USA) ultrasound system was used in frequency fusion mode and employing a 5.5–7.5 MHz trapezoidal multifrequency probe. Images were transferred to a frame grabber via a video Y/C link and images were digitized at a resolution of 768 × 576 pixels.
During the examination, unavoidable movements take place thus changing the relative position between the transducer and the artery. Therefore, expert intervention is sometimes required to control the image quality by readjusting the orientation of the transducer to keep visible borders as sharp as possible. Both, motion artifacts and successive readjustments may induce changes in image quality as well as changes on extraluminal structures along the sequence. All these factors have to be handled appropriately in the postprocessing stage if the computerized analysis has to be used on a routine basis.