
68
.pdf
G.V. Ayzel et al.
meteorological stations and stations ceased to exist in the first half of the 1990 year. That is way in this
work we used the database of indirect water level observations as well as climate reanalysis database.
Figure 1 - Satellite image of theAral Sea in July 2014 (left), satellite image of the
SmallAral inAugust 2014 (right)
The main goal of this work is to research |
which is located in arid conditions by using the |
the possibility of modeling the dynamic |
data of indirect measurements of open sources |
changeabilities of the water volume of basin, |
(Fig. 2). |
Figure 2 – Study area: the SmallAral Sea and theAral Sea basin
Key modeling concept has based on implementation of a simple Decision Tree model in case of regression task (Ayzel, 2017; Breiman et al., 1984).
Typical Decision Tree model is a «white box» consists of the range of boolean classifiers which split our samples to tiny «leaf» nodes where all samples
71

Evaluation of dynamic change of water level of the small aral sea based on data of open sources
constantly refers to the one target value (Hastie et al., 2005). Single tree-based implementation of Decision Tree is algorithm faced with the case of overfitting and robustness lack that lead to limited using in real world examples (Freund, Schapire, 1997). In our work, we used three cutting-edge machinelearning techniques based on ensemble approach to predictions: Random Forest, Extra Trees and Gradient Boosting (Ayzel, 2017; Mason et al., 2000; Zhang,Hsieh,2017).Allofthemarebasedongroup of simple Decision Tree models and provide useful trick such bagging, bootstraping, prunning etc. that totally reduce overfitting and make our models suitable to provide robust dynamics (Friedman, 2001; Kohavi, 1995; Schapire, Freund, 2012).
Materials and methods
The research material has selected as the analysis period from 2002 to 2014, all daily (reanalysis) and decadal (water level) measurements have taken to monthly values. Below is given more specific description of used the data:
1.Sea level variability based on satellite altimetry data from 1992 to 2014 (decadal values), DAHITI project (Schwatke et al., 2015);
2.River runoff of Syrdarya river (gauging station Kazaly), historical monthly values from 19791986, Global Runoff Data Center (GRDC) (www. bafg.de);
3.Atmospheric forcing: reanalysis Era-Interim, resolution 1x1 degree, daily values (Deeetal., 2011).
Ahypsometric relationship between the sea surfacelayerandthewatervolumewasobtainedforthe entire range of sea level variability according to the
DAHITI data for the period under research based on adetailedbathymetricmapoftheSmallAral(Izhitskiy et al., 2016b).
As a model linking the average monthly rates of fluctuations in climatic characteristics with the dynamics changeability of river runoff values (Ayzel and Izhitsky, 2016), a regression model of solving trees was chosen, which in general is a nonparametric model of machine learning - a simple model of the “white box” solutions, which can be described by a set of simple Boolean functions.Advantages of the regression model of decision trees are: interpretability,quicklearning,hightoleranceforincomplete data. As shortcomings, usually distinguished: high ability to retrain, instability with the dominance of one decisive class, the difficulty of finding the exact structure of the tree.
Results and discussion
Based on the described method, two statistical models were developed: a model which shows dynamic changeability of water level corresponding changes of water volume of Aral Sea and the variability forecast of the water volume of the Small Aral. In the first model, the predictors used air and precipitation values for the current and previous six months averaged over the entireAral Sea basin.The model was trained on the period from July 1979 to December 1985 according to the monthly values of theriverrunoffatthegaugingstationKazaly(Figure 3). As a result, it was used to simulate river runoff values for the period from January 1986 to September 2015 - for this period, the actual measurements of the river runoff were not available (Fig. 4).
Figure 3- Training of the model of river runoff formation for the period from July 1979 to December 1985
72
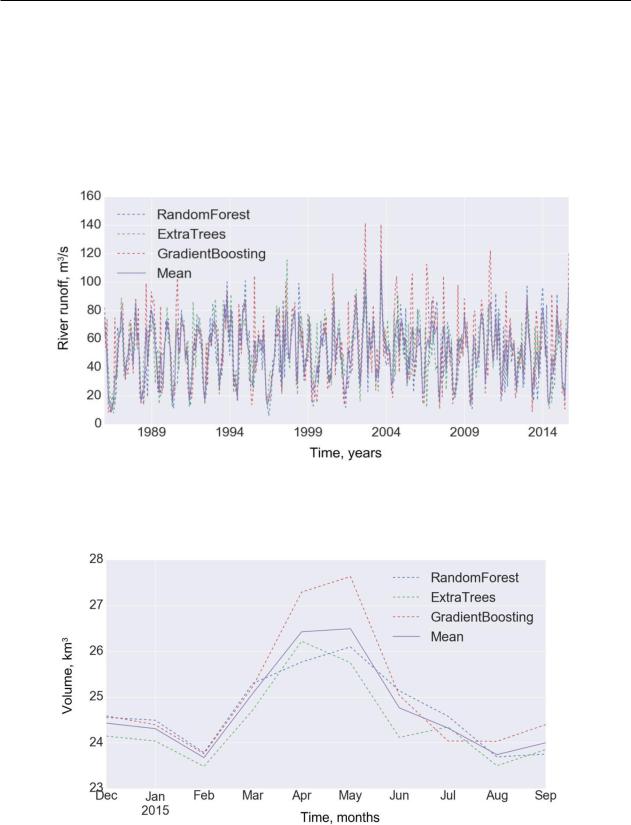
G.V. Ayzel et al.
These data, together with reanalysis data (air temperature, wind speed, precipitation) were used to train the second statistical model for predicting
Small Aral volume fluctuations on satellite altimetry data for the period from October 1992 to November 2014 (Fig. 5).
In conclusion, a modelling was made for the monthly values of the SmallAral Sea water volume
for 2015 (Figure 6). Analysis of the significance of the signs showed that the parameters of mean temperature, precipitation amount and total evaporation are most significant for the results of constructing the model of decision trees, so only they were chosen as changeability of the final model - this made themodellesssusceptibletonoise(robust),andalso minimized the risk of retraining.
Figure 4 - Simulation of monthly river runoff values for the period from January 1986 to September 2015
Figure 5 - Training model for forecasting the water volume of the SmallAral Sea, the period from October 1992 to November 2014
73

Evaluation of dynamic change of water level of the small aral sea based on data of open sources
Figure 6 - Modeling of monthly values of the water volume of the SmallAral Sea in 2015.
Annual river runoff of Syrdaria River in the lower reaches over a long period of time is characterized by instability, which is due to both natural and anthropogenic factors. At present, the influence of the anthropogenic factor on the natural ecological system is very large. One of the main negative factors is the irrational use of water infrastructure.
The Syrdarya River in the first half of the last century was significantly regulated. Since then, the largest monthly water discharge has been characteristic of the beginning of spring, the minimum - for the entire summer period.
This distribution of river runoff is due to large volumes of water for irrigation of agricultural fields in the upper and middle reaches. Spring floods in the lower reaches of the river. The Syrdarya usually begins in late March - early April.Then, in connection with the filling of overlying reservoirs and water intakes, a recession begins in April.
The lowest monthly water discharges (in some years - 6-10 m3/s) at the mouth of the river (Kazaly gauging station) has observed in the summer months, when irrigation of agricultural land is in full swing.
Water discharge, m3/s
2000
1800
1600
1400
1200
1000
800
600
400
200
0
1 |
2 |
3 |
4 |
5 |
6 |
7 |
8 |
9 |
10 |
11 |
12 |
Month
2006
2007
2008
2009
2010
2015
2016
Figure 7 – The annual runoff distribution of the lower reaches of the Syrdarya river
74
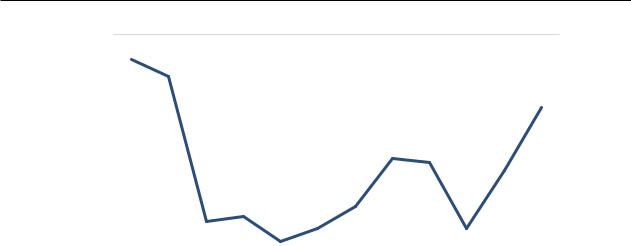
G.V. Ayzel et al.
Water discharge, m3/s
500
450 |
|
|
|
|
|
|
|
|
Kazaly |
|
|
|
|
|
|
|
|
|
|
|
|
|
|
|
|
||
|
|
|
|
|
|
|
|
|
|
|
|
|
|
400 |
|
|
|
|
|
|
|
|
|
|
|
|
|
|
|
|
|
|
|
|
|
|
|
|
|
|
|
350 |
|
|
|
|
|
|
|
|
|
|
|
|
|
|
|
|
|
|
|
|
|
|
|
|
|
|
|
300 |
|
|
|
|
|
|
|
|
|
|
|
|
|
|
|
|
|
|
|
|
|
|
|
|
|
|
|
250 |
|
|
|
|
|
|
|
|
|
|
|
|
|
|
|
|
|
|
|
|
|
|
|
|
|
|
|
200 |
|
|
|
|
|
|
|
|
|
|
|
|
|
|
|
|
|
|
|
|
|
|
|
|
|
|
|
150 |
|
|
|
|
|
|
|
|
|
|
|
|
|
|
|
|
|
|
|
|
|
|
|
|
|
|
|
100 |
|
|
|
|
|
|
|
|
|
|
|
|
|
|
|
|
|
|
|
|
|
|
|
|
|
|
|
50 |
|
|
|
|
|
|
|
|
|
|
|
|
|
|
|
|
|
|
|
|
|
|
|
|
|
|
|
0 |
|
|
|
|
|
|
|
|
|
|
|
|
|
|
|
|
|
|
|
|
|
|
|
|
|
|
|
1 |
2 |
3 |
4 |
5 |
6 |
7 |
8 |
9 |
10 |
11 |
12 |
Month
Figure 8 – Monthly distribution of runoff of the lower reaches of the Syrdarya – Kazaly gauging station for 2016 year
The dynamics of the average monthly annual runoff in the lower Syrdaria River is following: the loss of river runoff in the section from Kazaly to Karatereni (86 km), depending on the month, ranged from55to358m3.Suchadifferencebetweenrunoff at the Kazaly and Karateren hydroelectric facilities is associated with the filling of lakes in the coastal zone. Losses associated with the transformation of runoff in the riverbed and floodplain of the river are to a large extent recoverable, if we consider not a short time interval, but a long period during which the water of detention in the section can drain through the lower closure target. Along with such losses, there are irretrievable losses of water in the area under consideration for filling numerous floodplain lakes and old lakes that do not communicate with the river, infiltration into the soil in the floodplain and evaporation.
Lower Syrdaria River in the second half of the last century, acutely felt a shortage of water, and about 30 years ago, runoff at its mouth decreased almost to zero. However, at the end of the 80s on the territory of the former USSR, people’s views on the state of the environment changed dramatically, and the Syrdaria River delta became more careful about waterresources.Asaresult,alreadyintheearly90s, water along the river. The Syrdaria River began to flow regularly into the northern part of theAral Sea. Although the volume of this water was about 2-4 times less than the amount of natural runoff (14.9 km3), it was enough to flood a significant part of the modern “SmallAral” and there the desalination process began.
Conclusion
As a result, it should be noted that despite the serious shortage of these direct measurements of the main components of the water balance, such as the sea surface level and river runoff, the proposed method makes it possible to accurately predict the volume of waters of the Small Aral on the basis of open databases. The introduction of the factor of influence of the Kokaral dam into the model will help improve the quality of the forecast. The proposed technique can be used to obtain initial conditions in hydrodynamic simulation experiments, as well as to calculate climatic scenarios for the development of the hydrological system of the Aral Sea. Directions of further studies of the dynamics of the water volume of the Small Aral Sea are seen in the use of episodic data of field measurements for verification and more detailed adjustment of the model used,
Syrdarya for the restoration of the values of natural water inflow into the SmallAral Sea.
Acknowledgment
This work was supported by the Russian Foundation for Basic Research, project 17-05-01175 A, and the Science Committee of the Ministry of Education and Science of the Republic of Kazakhstan in the framework of the scientific Project №
AP05134202 «The current ecological state of endorheic lakes of the arid climate zone and perspectivesoftheirsustainableuse:thecaseoftheAralsea residual basins».
75

Evaluation of dynamic change of water level of the small aral sea based on data of open sources
References
Ayzel G.V. Use of machine learning techniques for modeling of snow depth. Ice and Snow. 2017;57(1):34-44. (In Russ.)
DOI:10.15356/2076-6734-2017-1-34-44
Ayzel G.V., Izhitskiy А.S. (2016). Issledobanie dinamiki urovnyavodi Aralskogo moray podannym distancionnogo zondiro vanya I klimaticheskogo reanalaza //Vodnye resursy: izuchenye I upravlenye (limnogologicheskaya shkola-praktika), [Dynamics of the Aral Sea Mountaineering in the range of remote sensing and climatic reanalysis] Water resources: learning and governance (limnological school-practice). Тom 2. P.p 189-19
Breiman L., Friedman J., Stone C.J., Olshen R.A. Classification and regression trees. CRC press, 1984: 360 p.
Dee, D.P. et al. The ERA-Interim reanalysis: configuration and performance of the data assimilation system. Quarterly Journal of the Royal Meteorological Society, 137: 553–597. doi: 10.1002/qj.828
Freund Y., Schapire R.E.Adecision-theoretic generalization of on-line learning and an application to boosting. Journ. of computer and system sciences. 1997, 55 (1): 119–139. doi: 10.1007/3-540-59119-2_166.
Friedman J.H. Greedy function approximation: a gradient boosting machine.Annals of Statistics. 2001, 29(5): 1189–1232. Hastie T., Tibshirani R., Friedman J., Franklin J. The elements of statistical learning: data mining, inference and prediction.
Springler, 2005: 758 p.
IzhitskiyA.S.,Ayzel G.V., Zavialov P.O., KurbaniyazovA.K. Estimation of theAral Sea state predictability based on the open data sources and the unique field observations. Geophysical ResearchAbstracts. Vol.18, EGU2016-6131, 2016a.
Izhitskiy,A.S. et al. Present state of theAral Sea: diverging physical and biological characteristics of the residual basins. Scientific Reports, 6, 23906 (2016b) doi:10.1038/srep23906
KohaviR.Astudyofcross-validationandbootstrapforaccuracyestimationandmodelselection.Intern.JointConf.onArtificial Intelligence (Ijcai). 1995, 14 (2): 1137–1145.
Mason, L., Baxter, J., Bartlett, P. L., & Frean, M. R. (2000). Boosting algorithms as gradient descent. In Advances in neural information processing systems (pp. 512-518).
Monthly runoff data. The Global Runoff Data Centre, 56068 Koblenz, Germany. www.bafg.de
Sambaev N.S. Modern hydroecological condition of the lower runoff of the Syrdarya river and use of its resource resources // Astrakhan bulletin of ecological education, No. 2 (40) 2017. p. 50-55.
Schapire R.E., FreundY. Boosting: Foundations and algorithms. MIT press, 2012: 528 p.
Schwatke, C. et al. DAHITI - an innovative approach for estimating water level time series over inland waters using multimission satellite altimetry, Hydrology and Earth System Sciences, 2015, 19, 4345-4364,
Zavialov, Peter O. Physical oceanography of the dyingAral Sea. Springer Science & Business Media, 2007. Zhang, H., Si, S., and Hsieh, C.-J.: GPU-acceleration for Large-scale Tree Boosting, http://arxiv.org/abs/1706.08359, 2017.
Литература
Айзель Г.В. Использование методов машинного обучения для моделирования глубины снега. Лед и снег. 2017; 57 (1):
34-44. DOI: 10.15356 / 2076-6734-2017-1-34-44.
Айзель Г.В., Ижицкий А.С. (2016). Исследования динамики уровня Аральского Моря по данным дистанционного зондирования и климатического реанализа // Водные ресурсы: Изучение и управление (Лимгол. Школа-практика). Том 2. – С. 189-19.
Брейман Л., Фридман Дж., Стоун С.Дж., Ольшен Р.А. Деревья классификации и регрессии. CRC пресс, 1984: 360 с. Ди,Д.П.идругие.ПовторныйанализERA-Interim:конфигурацияипроизводительностьсистемыассимиляцииданных.
Ежеквартальный журнал Королевского метеорологического общества, 137: 553–597. doi: 10.1002 / qj.828
Фрейнд Ю., Щайпре Р.И. Теоретическое обобщение онлайн обучения и приложение для повышения. Журнал компьютерных и системных наук. 1997, 55 (1): 119–139. doi: 10.1007 / 3-540-59119-2_166.
Фридман Дж. Х. Приближение жадных функций: машина повышения градиента // Летопись статистики. 2001, 29 (5): 1189–1232.
Хасти Т., Тибширани Р., Фридман Дж., Франклин Дж. Элементы статистического обучения: интеллектуальный анализ данных, логический вывод и прогнозирование. – Спринглер, 2005: 758 с.
Ижицкий А.С., Айзель Г.В., Завьялов П.О., Курбаниязов А.К. Оценка предсказуемости состояния Аральского моря на основе открытых источников данных и уникальных полевых наблюдений. Рефераты геофизических исследований. Том 18,
EGU2016-6131, 2016a.
Ижицкий, А.С. и другие. Современное состояние Аральского моря: различные физические и биологические характеристики остаточных бассейнов. Научный отчет, 6, 23906 (2016b) doi: 10.1038 / srep23906
Кохави Р. Исследование перекрестной проверки и начальной загрузки для оценки точности и выбора модели. Междун. Совмест. конф. по искусственному интеллекту. 1995, 14 (2): 1137–1145.
Мейсон Л., Бакстер Дж., Бартлетт П.Л. & Фрэйн M.Р. (2000). Повышающие алгоритмы как градиентный спуск. в Достижения в нейронных системах обработки информации (стр. 512-518).
Ежемесячные данные по стоку. Глобальный центр данных по стоку, 56068 Кобленц, Германия. www.bafg.de.
Самбаев Н.С. Современное гидроэкологическое состояние нижнего течения реки Сырдарья и использование ее ресурсов стока // Астраханский вестник экологического образования. – № 2 (40) 2017. – С. 50-55.
76

G.V. Ayzel et al.
Щапрай Р.Э., Фрейнд Й. Стимулирование: основы и алгоритмы. MIT пресс, 2012: 528 с.
Шватке C. и др DAHITI - инновационный подход для оценки временных рядов уровня воды над внутренними водами с использованием многоцелевой спутниковой альтиметрии. Гидрология и науки о Земле. 2015, 19, 4345-4364.
Завьялов Петр Олегович. Физическая океанография умирающего Аральского моря. Спрингер Наука и Бизнес медиа, 2007. Чжан Х., Си, С. и Хси К.-Дж. GPU-ускорение для крупномасштабного ускорения деревьев, http://arxiv.org/ abs/1706.08359, 2017.
77
4-бөлім
РЕКРЕАЦИЯЛЫҚ ГЕОГРАФИЯ ЖӘНЕ ТУРИЗМ
Section 4
RECREATION GEOGRAPHY
AND TOURISM
Раздел 4
РЕКРЕАЦИОННАЯ ГЕОГРАФИЯ И ТУРИЗМ
ISSN 1563-0234, eISSN 2663-0397 |
Хабаршы. География сериясы. №2 (57) 2020 |
https://bulletin-orientalism.kaznu.kz |
ҒТАМP71.37.75 |
https://doi.org/10.26577/JGEM.2020.v57.i2.07 |
А.С. Актымбаева,А.Н.Бекен
, У.Т.Кошкимбаева
, А.Ж. Сапиева
Әл-Фараби атындағы Қазақ ұлттық университеті, Қазақстан, Алматы қ. *Корреспонденттік автор – А.Н. Бекен
e-mail: botabeken@gmail.com
ТУPИСТІК-PEКPEАЦИЯЛЫҚ PEСУPСТАP ТУPИСТІКPEКPEАЦИЯЛЫҚ ӘЛEУEТТІҢ НEГІЗІ PEТІНДE: ШЫҒЫС ҚАЗАҚСТАН ОБЛЫСЫ МЫСАЛЫНДА
Осы ғылыми мақалада туристік-рекреациялық салада туындаған терминологиялық проблемаларқарастырылады.Олардыңбірі«туристікресурс»және«туристікәлеует»терминдерінің арасындағы семантикалық айырмашылықтармен байланысты. Сондай-ақ отандық және шетелдік зерттеушілердің «туристік ресурстарды» және «туристік әлеуетті» бағалаудың әртүрлі тәсілдеріне талдау жүргізілді. Қарастырылып отырған мәселелердің бірі табиғи туристік-рекреациялық ресурстарды туристік-рекреациялық әлеуеттің негізі ретінде зерттеу болып табылады. Аумақтың рекреациялық әлеуетін перспективалы аумақтық рекреациялық жүйелердегі рекреация мен туризмді дамытуда объективті және субъективті алғышарттардың жиынтығы ретінде қарастыру қажет екендігі белгіленді. Сонымен қатар, өңірдің туристік және рекреациялық әлеуеті туристікрекреациялық жүйелерді жобалаудың, орналастырудың және дамытуды оңтайландырудың, сондай-ақ олардың меншікті ресурстарының құндылығы мен олардың үйлесімділігіне, туризм мен рекреацияның тұрақты дамуына сүйене отырып, олардың негізгі функцияларын анықтаудың маңызды бөлігі болып табылатыны анықталды. Осылайша, Шығыс Қазақстан облысы (ШҚО) мысалында табиғи факторлардың рекреациялық қызметтің объектілері мен бағыттарына тәуелділігі көрсетілген. Бұдан басқа, осы ғылыми мақала шеңберінде Шығыс Қазақстан облысы бойынша туризмді дамытудың табиғи алғышарттарына бағалау жүргізілді және аттрактивтілік дәрежесі бойынша ландшафттық түрлердің классификациясы талданды және осы өңірде туризмді дамытудың перспективалық бағыттары анықталды. Зерттеудің қорытынды нәтижесі ретінде ғалымдар мен зерттеушілердің түрлі әдістемелерін қолдану негізінде Шығыс Қазақстан облысының аумағын табиғи ландшафттардың аттрактивтілік дәрежесі бойынша аудандастыру картасы жасалды.
Түйін сөздep: туpистік peсуpс, туpистік әлeуeт, табиғи туpистік-peкpeациялық peсуpстаp, туpистік-peкpeациялық әлeуeт, Шығыс Қазақстан облысы.
A.S. Aktymbayeva, A.N. Beken, U.T. Koshkimbayeva, A.Zh. Sapiyeva
Al-Farabi Kazakh National University, Kazakhstan, Almaty *Сorrespondent author – A.Zh. Sapiyeva
e-mail: botabeken@gmail.com
Tourist and recreational resources as the basis of tourist and recreational potential: the case of East Kazakhstan oblast
This paper discusses terminological problems that have arisen in tourist-recreational sphere. One of them is related to the semantic differences between terms “tourist resource” and “tourist potential”. There was also an analysis of various ways to assess the “tourist resources” and “tourist potential” of domestic and foreign researchers. One of the issues under consideration is the study of natural tourist and recreational resources as the basis of tourist and recreational potential. It was noted that the recreational potential of territory should be considered as a set of objective and subjective prerequisites for the development of recreation and tourism in promising territorial recreational systems. Moreover, it was found that the tourism and recreation potential of the region is an important part of the design, placement and optimization of development of tourism and recreation systems, as well as determining their main functions, based on the value of their own resources and their combination, sustainable development of tourism and recreation. Thus, dependence of natural factors on objects and recreational activities is shown on the case of East Kazakhstan oblast (EKO). In addition, within the framework of this paper, the East Kazakhstan oblast region has assessed natural prerequisites for tourism development and analyzed
80 |
© 2020 Al-Farabi Kazakh National University |